Train AI on Repetitive Data? Sure, Cheap, Good Enough, But, But, But
August 8, 2024
We already know that AI algorithms are only as smart as the data that trains them. If the data models are polluted with bias such as racism and sexism, the algorithms will deliver polluted results. We’ve also learned that while some of these models are biased because of innocent ignorance. Nature has revealed that AI algorithms have yet another weakness: “AI Models Collapse When Trained On Recursively Generated Data.”
Generative text AI aka large language models (LLMs) are already changing the global landscape. While generative AI is still in its infancy, AI developers are already designing the next generation. There’s one big problem: LLMs. The first versions of Chat GPT were trained on data models that scrapped content from the Internet. GPT continues to train on models using the same scrapping methods, but it’s creating a problem:
“If the training data of most future models are also scraped from the web, then they will inevitably train on data produced by their predecessors. In this paper, we investigate what happens when text produced by, for example, a version of GPT forms most of the training dataset of following models. What happens to GPT generations GPT-{n} as n increases? We discover that indiscriminately learning from data produced by other models causes ‘model collapse’—a degenerative process whereby, over time, models forget the true underlying data distribution, even in the absence of a shift in the distribution over time.”
The generative AI algorithms are learning from copies of copies. Over time the integrity of the information fails. The research team behind the Nature paper discovered that model collapse is inevitable when with the most ideal conditions. The team did discover two possibilities to explain model collapse: intentional data poisoning and task-free continual learning. Those don’t explain recursive data collapse with models free of those events.
The team concluded that the best way for generative text AI algorithms to learn was continual interaction learning from humans. In other words, the LLMs need constant, new information created by humans to replicate their behavior. It’s simple logic when you think about it.
Whitney Grace, August 8, 2024
Publishers Perplexed with Perplexity
August 7, 2024
In an about-face, reports Engadget, “Perplexity Will Put Ads in it’s AI Search Engine and Share Revenue with Publishers.” The ads part we learned about in April, but this revenue sharing bit is new. Is it a response to recent accusations of unauthorized scraping and plagiarism? Nah, the firm insists, the timing is just a coincidence. While Perplexity won’t reveal how much of the pie they will share with publishers, the company’s chief business officer Dmitry Shevelenko described it as a “meaningful double-digit percentage.” Engadget Senior Editor Pranav Dixit writes:
“‘[Our revenue share] is certainly a lot more than Google’s revenue share with publishers, which is zero,’ Shevelenko said. ‘The idea here is that we’re making a long-term commitment. If we’re successful, publishers will also be able to generate this ancillary revenue stream.’ Perplexity, he pointed out, was the first AI-powered search engine to include citations to sources when it launched in August 2022.”
Defensive much? Dixit reminds us Perplexity redesigned that interface to feature citations more prominently after Forbes criticized it in June.
Several AI companies now have deals to pay major publishers for permission to scrape their data and feed it to their AI models. But Perplexity does not train its own models, so it is taking a piece-work approach. It will also connect advertisements to searches. We learn:
“‘Perplexity’s revenue-sharing program, however, is different: instead of writing publishers large checks, Perplexity plans to share revenue each time the search engine uses their content in one of its AI-generated answers. The search engine has a ‘Related’ section at the bottom of each answer that currently shows follow-up questions that users can ask the engine. When the program rolls out, Perplexity plans to let brands pay to show specific follow-up questions in this section. Shevelenko told Engadget that the company is also exploring more ad formats such as showing a video unit at the top of the page. ‘The core idea is that we run ads for brands that are targeted to certain categories of query,’ he said.”
The write-up points out the firm may have a tough time breaking into an online ad business dominated by Google and Meta. Will publishers hand over their content in the hope Perplexity is on the right track? Launched in 2022, the company is based in San Francisco.
Cynthia Murrell, August 7, 2024
AI Research: A New and Slippery Cost Center for the Google
August 7, 2024
This essay is the work of a dumb humanoid. No smart software required.
A week or so ago, I read “Scaling Exponents Across Parameterizations and Optimizers.” The write up made crystal clear that Google’s DeepMind can cook up a test, throw bodies at it, and generate a bit of “gray” literature. The objective, in my opinion, was three-fold. [1] The paper makes clear that DeepMind is thinking about its smart software’s weaknesses and wants to figure out what to do about them. And [2] DeepMind wants to keep up the flow of PR – Marketing which says, “We are really the Big Dogs in this stuff. Good luck catching up with the DeepMind deep researchers.” Note: The third item appears after the numbers.
I think the paper reveals a third and unintended consequence. This issue is made more tangible by an entity named 152334H and captured in “Calculating the Cost of a Google DeepMind Paper.” (Oh, 152334 is a deep blue black color if anyone cares.)
That write up presents calculations supporting this assertion:
How to burn US$10,000,000 on an arXiv preprint
The write up included this table presenting the costs to replicate what the xx Googlers and DeepMinders did to produce the ArXiv gray paper:
Notice, please, that the estimate is nearly $13 million. Anyone want to verify the Google results? What am I hearing? Crickets.
The gray paper’s 11 authors had to run the draft by review leadership and a lawyer or two. Once okayed, the document was converted to the arXiv format, and we the findings to improve our understanding of how much work goes into the achievements of the quantumly supreme Google.
Thijs number of $12 million and change brings me to item [3]. The paper illustrates why Google has a tough time controlling its costs. The paper is not “marketing,” because it is R&D. Some of the expense can be shuffled around. But in my book, the research is overhead, but it is not counted like the costs of cubicles for administrative assistants. It is science; it is a cost of doing business. Suck it up, you buttercups, in accounting.
The write up illustrates why Google needs as much money as it can possibly grab. These costs which are not really nice, tidy costs have to be covered. With more than 150,000 people working on projects, the costs of “gray” papers is a trigger for more costs. The compute time has to be paid for. Hello, cloud customers. The “thinking time” has to be paid for because coming up with great research is open ended and may take weeks, months, or years. One could not rush Einstein. One cannot rush Google wizards in the AI realm either.
The point of this blog post is to create a bit of sympathy for the professionals in Google’s accounting department. Those folks have a tough job figuring out how to cut costs. One cannot prevent 11 people from burning through computer time. The costs just hockey stick. Consequently the quantumly supreme professionals involved in Google cost control look for simpler, more comprehensible ways to generate sufficient cash to cover what are essentially “surprise” costs. These tools include magic wand behavior over payments to creators, smart commission tables to compensate advertising partners, and demands for more efficiency from Googlers who are not thinking big thoughts about big AI topics.
Net net: Have some awareness of how tough it is to be quantumly supreme. One has to keep the PR and Marketing messaging on track. One has to notch breakthroughs, insights, and innovations. What about that glue on the pizza thing? Answer: What?
Stephen E Arnold, August 7, 2024
Old Problem, New Consequences: AI and Data Quality
August 6, 2024
This essay is the work of a dinobaby. Unlike some folks, no smart software improved my native ineptness.
Grab a business card from several years ago. Just for laughs send an email to the address on the card or dial one of the numbers printed on it. What happens? Does the email bounce? Does the person you called answer? In my experience, the business cards I have gathered at conferences in 2021 are useless. The number rings in space or a non-human voice says, “The number has been disconnected.” The emails go into a black hole. I would, based on my experience, peg the 100 random cards I had one of my colleagues pull from the files that work at fewer than 30 percent. In 24 months, 70 percent of the data are invalid. An optimist would say, “You have 30 people you can contact.” A pessimist would say, “Wow, you lost 70 contacts.” A 20-something whiz kid at one of the big time AI companies would say, “Good enough.”
An automated data factory purports to manufacture squares. What does it do? Triangles are good enough and close enough for horseshoes. Does the factory call the triangles squares? Of course, it does. Thanks, MSFT Copilot. Security is Job One today I hear.
I read “Data Quality: The Unseen Villain of Machine Learning.” The write up states:
Too often, data scientists are the people hired to “build machine learning models and analyze data,” but bad data prevents them from doing anything of the sort. Organizations put so much effort and attention into getting access to this data, but nobody thinks to check if the data going “in” to the model is usable. If the input data is flawed, the output models and analyses will be too.
Okay, that’s a reasonable statement. But this passage strikes me as a bit orthogonal to the observations I have made:
It is estimated that data scientists spend between 60 and 80 percent of their time ensuring data is cleansed, in order for their project outcomes to be reliable. This cleaning process can involve guessing the meaning of data and inferring gaps, and they may inadvertently discard potentially valuable data from their models. The outcome is frustrating and inefficient as this dirty data prevents data scientists from doing the valuable part of their job: solving business problems. This massive, often invisible cost slows projects and reduces their outcomes.
The painful reality, in my experience, consists of three factors:
- Data quality depends on the knowledge and resources available to a subject matter expert. A data quality expert might define quality as consistent data; that is, the name field has a name. The SME figures out if the data are in line with other data and what’s is off base.
- The time required to “ensure” data quality is rarely available. There are interruptions, Zooms, and automated calendars that ping a person for a meeting. Data quality is easily killed by time suffocation.
- The volume of data begs for automated procedures and, of course, AI. The problem is that the range of errors related to validity is sufficiently broad to allow “flawed” data to enter a systems. Good enough creates interesting consequences.
The write up says:
Data quality shouldn’t be a case of waiting for an issue to occur in production and then scrambling to fix it. Data should be constantly tested, wherever it lives, against an ever-expanding pool of known problems. All stakeholders should contribute and all data must have clear, well-defined data owners. So, when a data scientist is asked what they do, they can finally say: build machine learning models and analyze data.
This statement makes clear why flawed data remain flawed. The fix, according to some, is synthetic data. Are these data of high quality? It depends on what one means by “quality.” Today the benchmark is good enough. Good enough produces outputs that are not. But who knows? Not the harried person looking for something, anything, to put in a presentation, journal article, or podcast.
Stephen E Arnold, August 6, 2024
MBAs Gone Wild: Assertions, Animation & Antics
August 5, 2024
Author’s note: Poor WordPress in the Safari browser is having a very bad day. Quotes from the cited McKinsey document appear against a weird blue background. My cheerful little dinosaur disappeared. And I could not figure out how to claim that AI did not help me with this essay. Just a heads up.
Holed up in rural Illinois, I had time to read the mid-July McKinsey & Company document “McKinsey Technology Trends Outlook 2024.” Imagine a group of well-groomed, top-flight, smooth talking “experts” with degrees from fancy schools filming one of those MBA group brainstorming sessions. Take the transcript, add motion graphics, and give audio sweetening to hot buzzwords. I think this would go viral among would-be consultants, clients facing the cloud of unknowing about the future. and those who manifest the Peter Principle. Viral winner! From my point of view, smart software is going to be integrated into most technologies and is, therefore, the trend. People may lose money, but applied AI is going to be with most companies for a long, long time.
The report boils down the current business climate to a few factors. Yes, when faced with exceptionally complex problems, boil those suckers down. Render them so only the tasty sales part remains. Thus, today’s businesss challenges become:
Generative AI (gen AI) has been a standout trend since 2022, with the extraordinary uptick in interest and investment in this technology unlocking innovative possibilities across interconnected trends such as robotics and immersive reality. While the macroeconomic environment with elevated interest rates has affected equity capital investment and hiring, underlying indicators—including optimism, innovation, and longer-term talent needs—reflect a positive long-term trajectory in the 15 technology trends we analyzed.
The data for the report come from inputs from about 100 people, not counting the people who converted the inputs into the live-action report. Move your mouse from one of the 15 “trends” to another. You will see the graphic display colored balls of different sizes. Yep, tiny and tinier balls and a few big balls tossed in.
I don’t have the energy to take each trend and offer a comment. Please, navigate to the original document and review it at your leisure. I can, however, select three trends and offer an observation or two about this very tiny ball selection.
Before sharing those three trends, I want to provide some context. First, the data gathered appear to be subjective and similar to the dorm outputs of MBA students working on a group project. Second, there is no reference to the thought process itself which when applied to a real world problem like boosting sales for opioids. It is the thought process that leads to revenues from consulting that counts.
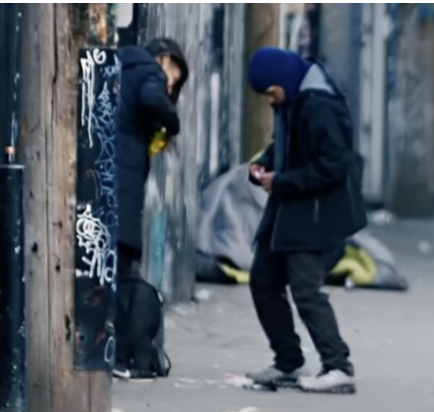
Third, McKinsey’s pool of 100 thought leaders seems fixated on two things:
gen AI and electrification and renewables.
But is that statement comprised of three things? [1] AI, [2] electrification, and [3] renewables? Because AI is a greedy consumer of electricity, I think I can see some connection between AI and renewable, but the “electrification” I think about is President Roosevelt’s creating in 1935 the Rural Electrification Administration. Dinobabies can be such nit pickers.
Let’s tackle the electrification point before I get to the real subject of the report, AI in assorted forms and applications. When McKinsey talks about electrification and renewables, McKinsey means:
The electrification and renewables trend encompasses the entire energy production, storage, and distribution value chain. Technologies include renewable sources, such as solar and wind power; clean firm-energy sources, such as nuclear and hydrogen, sustainable fuels, and bioenergy; and energy storage and distribution solutions such as long-duration battery systems and smart grids.In 2019, the interest score for Electrification and renewables was 0.52 on a scale from 0 to 1, where 0 is low and 1 is high. The innovation score was 0.29 on the same scale. The adoption rate was scored at 3. The investment in 2019 was 160 on a scale from 1 to 5, with 1 defined as “frontier innovation” and 5 defined as “fully scaled.” The investment was 160 billion dollars. By 2023, the interest score for Electrification and renewables was 0.73. The innovation score was 0.36. The investment was 183 billion dollars. Job postings within this trend changed by 1 percent from 2022 to 2023.
Stop burning fossil fuels? Well, not quite. But the “save the whales” meme is embedded in the verbiage. Confused? That may be the point. What’s the fix? Hire McKinsey to help clarify your thinking.
AI plays the big gorilla in the monograph. The first expensive, hairy, yet promising aspect of smart software is replacing humans. The McKinsey report asserts:
Generative AI describes algorithms (such as ChatGPT) that take unstructured data as input (for example, natural language and images) to create new content, including audio, code, images, text, simulations, and videos. It can automate, augment, and accelerate work by tapping into unstructured mixed-modality data sets to generate new content in various forms.
Yep, smart software can produce reports like this one: Faster, cheaper, and good enough. Just think of the reports the team can do.
The third trend I want to address is digital trust and cyber security. Now the cyber crime world is a relatively specialized one. We know from the CrowdStrike misstep that experts in cyber security can wreck havoc on a global scale. Furthermore, we know that there are hundreds of cyber security outfits offering smart software, threat intelligence, and very specialized technical services to protect their clients. But McKinsey appears to imply that its band of 100 trend identifiers are hip to this. Here’s what the dorm-room btrainstormers output:
The digital trust and cybersecurity trend encompasses the technologies behind trust architectures and digital identity, cybersecurity, and Web3. These technologies enable organizations to build, scale, and maintain the trust of stakeholders.
Okay.
I want to mention that other trends range from blasting into space to software development appear in the list. What strikes me as a bit of an oversight is that smart software is going to be woven into the fabric of the other trends. What? Well, software is going to surf on AI outputs. And big boy rockets, not the duds like the Seattle outfit produces, use assorted smart algorithms to keep the system from burning up or exploding… most of the time. Not perfect, but better, faster, and cheaper than CalTech grads solving equations and rigging cybernetics with wire and a soldering iron.
Net net: This trend report is a sales document. Its purpose is to cause an organization familiar with McKinsey and the organization’s own shortcomings to hire McKinsey to help out with these big problems. The data source is the dorm room. The analysts are cherry picked. The tone is quasi-authoritative. I have no problem with marketing material. In fact, I don’t have a problem with the McKinsey-generated list of trends. That’s what McKinsey does. What the firm does not do is to think about the downstream consequences of their recommendations. How do I know this? Returning from a lunch with some friends in rural Illinois, I spotted two opioid addicts doing the droop.
Stephen E Arnold, August 5, 2024
The Big Battle: Another WWF Show Piece for AI
August 2, 2024
This essay is the work of a dumb humanoid. No smart software required.
The Zuck believes in open source. It is like Linux. Boom. Market share. OpenAI believes in closed source (for now). Snap. You have to pay to get the good stuff. The argument about proprietary versus open source has been plodding along like Russia’s special operation for a long time. A typical response, in my opinion, is that open source is great because it allows a corporate interest to get cheap traction. Then with a surgical or not-so-surgical move, the big outfit co-opts the open source project. Boom. Semi-open source with a price tag becomes a competitive advantage. Proprietary software can be given away, licensed, or made available by subscription. Open source creates opportunities for training, special services, and feeling good about the community. But in the modern world of high-technology feeling good comes with sustainable flows of revenue and opportunities to raise prices faster than the local grocery store.
Where does open source software come from? Many students demonstrate their value by coding something useful to another. Thanks, Open AI. Good enough.
I read “Consider the Llama: Are Closed Source AI Models Doomed?” The write up is good. It contains a passage which struck me as interesting; to wit:
OpenAI, Anthropic and the like—companies that sell access to AI models. These companies inherently require their products to be much better than open source in order to up-charge. They also don’t have some other product they sell that gets improved with better AI overall.
In my opinion, in the present business climate, the hope that a high-technology product gets better is an interesting one. The idea of continual improvement, however, is not part of the business culture of high-technology companies engaged in smart software. At this time, cooking up a model which can be used to streamline or otherwise enhance an existing activity is Job One. The first outfit to generate substantial revenue from artificial intelligence will have an advantage. That doesn’t mean the outfit won’t fail, but if one considers the requirements to play with a reasonable probability of winning the AI game, smart software costs money.
In the world of online, a company or open source foundation which delivers a product or service which attracts large numbers of users has an advantage. One “play” can shift the playing field, not just win the game. What’s going on at this time, in my opinion, is that those who understand the advantage of winning in the equivalent of a WWF (World Wide Wrestling) show piece is that it allows the “winner take all” or at least the “winner takes two-thirds” of the market.
Monopolies (real or imagined) with lots of money have an advantage. Open source smart software have to have money from somewhere; otherwise, the costs of producing a winning service drop. If a large outfit with cash goes open source, that is a bold chess move which other outfits cannot afford to take. The feel good, community aspect of a smart software solution that can be used in a large number of use cases is going to fade quickly when any money on the table is taken by users who neither contribute, pay for training, or hire great open source coders as consultants. Serious players just take the software, innovate, and lock up the benefits.
“Who would do this?” some might ask.
How about China, Russia, or some nation state not too interested in the Silicon Valley way? How about an entrepreneur in Armenia or one of the Stans who wants to create a novel product or service and charge for it? Sure, US-based services may host the product or service, but the actual big bucks flow to the outfit who keeps the technology “secret”?
At this time, US companies which make high-value software available for free to anyone who can connect to the Internet and download a file are not helping American business. You may disagree. But I know that there are quite a few organizations (commercial and governmental) who think the US approach to open source software is just plain dumb.
Wrapping up an important technology with do-goodism and mostly faux hand waving about the community creates two things:
- An advantage for commercial enterprises who want to thwart American technical influence
- Free intelligence for nation-states who would like nothing more than convert the US into a client republic.
I did a job for a bunch of venture people who were into the open source religion. The reality is that at this time an alleged monopoly like Google can use its money and control of information flows to cripple other outfits trying to train their systems. On the other hand, companies who just want AI to work may become captive to an enterprise software vendor who is also an alleged monopoly. The companies funded by this firm have little chance of producing sustainable revenue. The best exits will be gift wrapping the “innovation” and selling it to another group of smart software-hungry investors.
Does the world need dozens of smart software “big dogs”? The answer is, “No.” At this time, the US is encouraging companies to make great strides in smart software. These are taking place. However, the rest of the world is learning and may have little or no desire to follow the open source path to the big WWF face off in the US.
The smart software revolution is one example of how America’s technology policy does not operate in a way that will cause our adversaries to do anything but download, enhance, build on, and lock up increasingly smarter AI systems.
From my vantage point, it is too late to undo the damage the wildness of the last few years can be remediated. The big winners in open source are not the individual products. Like the WWF shows, the winner is the promoter. Very American and decidedly different from what those in other countries might expect or want. Money, control, and power are more important than the open source movement. Proprietary may be that group’s preferred approach. Open source is software created by computer science students to prove they can produce code that does something. The “real” smart software is quite different.
Stephen E Arnold, August 2, 2024
Survey Finds Two Thirds of us Believe Chatbots Are Conscious
August 2, 2024
Well this is enlightening. TechSpot reports, “Survey Shows Many People Believe AI Chatbots Like ChatGPT Are Conscious.” And by many, writer Rob Thubron means two-thirds of those surveyed by researchers at the University of Waterloo. Two-thirds! We suppose it is no surprise the general public has this misconception. After all, even an AI engineer was famously convinced his company’s creation was sentient. We learn:
“The survey asked 300 people in the US if they thought ChatGPT could have the capacity for consciousness and the ability to make plans, reason, feel emotions, etc. They were also asked how often they used OpenAI’s product. Participants had to rate ChatGPT responses on a scale of 1 to 100, where 100 would mean absolute confidence that ChatGPT was experiencing consciousness, and 1 absolute confidence it was not. The results showed that the more someone used ChatGPT, the more they were likely to believe it had some form of consciousness. ‘These results demonstrate the power of language,’ said Dr. Clara Colombatto, professor of psychology at Waterloo’s Arts faculty, ‘because a conversation alone can lead us to think that an agent that looks and works very differently from us can have a mind.’”
That is a good point. And these “agents” will only get more convincing even as more of us interact with them more often. It is encouraging that some schools are beginning to implement AI Literacy curricula. These programs include important topics like how to effectively work with AI, when to double-check its conclusions, and a rundown of ethical considerations. More to the point here, they give students a basic understanding of what is happening under the hood.
But it seems we need a push for adults to educate themselves, too. Even a basic understanding of machine learning and LLMs would help. It will take effort to thwart our natural tendency to anthropomorphize, which is reinforced by AI hype. That is important, because when we perceive AI to think and feel as we do, we change how we interact with it. The write-up notes:
“The study, published in the journal Neuroscience of Consciousness, states that this belief could impact people who interact with AI tools. On the one hand, it may strengthen social bonds and increase trust. But it may also lead to emotional dependence on the chatbots, reduce human interactions, and lead to an over-reliance on AI to make critical decisions.”
Soon we might even find ourselves catering to perceived needs of our software (or the actual goals of the firms that make them) instead of using them as inanimate tools. Is that a path we really want to go down? Is it too late to avoid it?
Cynthia Murrell, August 2, 2024
A Reliability Test for General-Purpose AI
August 1, 2024
A team of researchers has developed a valuable technique: “How to Assess a General-Purpose AI Model’s Reliability Before It’s Deployed.” The ScienceDaily article begins by defining foundation models—the huge, generalized deep-learning models that underpin generative AI like ChatGPT and DALL-E. We are reminded these tools often make mistakes, and that sometimes these mistakes can have serious consequences. (Think self-driving cars.) We learn:
“To help prevent such mistakes, researchers from MIT and the MIT-IBM Watson AI Lab developed a technique to estimate the reliability of foundation models before they are deployed to a specific task. They do this by considering a set of foundation models that are slightly different from one another. Then they use their algorithm to assess the consistency of the representations each model learns about the same test data point. If the representations are consistent, it means the model is reliable. When they compared their technique to state-of-the-art baseline methods, it was better at capturing the reliability of foundation models on a variety of downstream classification tasks. Someone could use this technique to decide if a model should be applied in a certain setting, without the need to test it on a real-world dataset. This could be especially useful when datasets may not be accessible due to privacy concerns, like in health care settings. In addition, the technique could be used to rank models based on reliability scores, enabling a user to select the best one for their task.”
Great! See the write-up for the technical details behind the technique. This breakthrough can help companies avoid mistakes before they launch their products. That is, if they elect to use it. Will organizations looking to use AI for cost cutting go through these processes? Sadly, we suspect that, if costs go down and lawsuits are few and far between, the AI is deemed good enough. But thanks for the suggestion, MIT.
Cynthia Murrell, August 1, 2024
Google and Its Smart Software: The Emotion Directed Use Case
July 31, 2024
This essay is the work of a dumb humanoid. No smart software required.
How different are the Googlers from those smack in the middle of a normal curve? Some evidence is provided to answer this question in the Ars Technica article “Outsourcing Emotion: The Horror of Google’s “Dear Sydney” AI Ad.” I did not see the advertisement. The volume of messages flooding through my channels each days has allowed me to develop what I call “ad blindness.” I don’t notice them; I don’t watch them; and I don’t care about the crazy content presentation which I struggle to understand.
A young person has to write a sympathy card. The smart software is encouraging to use the word “feel.” This is a word foreign to the individual who wants to work for big tech someday. Thanks, MSFT Copilot. Do you have your hands full with security issues today?
Ars Technica watches TV and the Olympics. The write up reports:
In it, a proud father seeks help writing a letter on behalf of his daughter, who is an aspiring runner and superfan of world-record-holding hurdler Sydney McLaughlin-Levrone. “I’m pretty good with words, but this has to be just right,” the father intones before asking Gemini to “Help my daughter write a letter telling Sydney how inspiring she is…” Gemini dutifully responds with a draft letter in which the LLM tells the runner, on behalf of the daughter, that she wants to be “just like you.”
What’s going on? The father wants to write something personal to his progeny. A Hallmark card may never be delivered from the US to France. The solution is an emessage. That makes sense. Essential services like delivering snail mail are like most major systems not working particularly well.
Ars Technica points out:
But I think the most offensive thing about the ad is what it implies about the kinds of human tasks Google sees AI replacing. Rather than using LLMs to automate tedious busywork or difficult research questions, “Dear Sydney” presents a world where Gemini can help us offload a heartwarming shared moment of connection with our children.
I find the article’s negative reaction to a Mad Ave-type of message play somewhat insensitive. Let’s look at this use of smart software from the point of view of a person who is at the right hand tail end of the normal distribution. The factors in this curve are compensation, cleverness as measured in a Google interview, and intelligence as determined by either what school a person attended, achievements when a person was in his or her teens, or solving one of the Courant Institute of Mathematical Sciences brain teasers. (These are shared at cocktail parties or over coffee. If you can’t answer, you pay the bill and never get invited back.)
Let’s run down the use of AI from this hypothetical right of loser viewpoint:
- What’s with this assumption that a Google-type person has experience with human interaction. Why not send a text even though your co-worker is at the next desk? Why waste time and brain cycles trying to emulate a Hallmark greeting card contractor’s phraseology. The use of AI is simply logical.
- Why criticize an alleged Googler or Googler-by-the-gig for using the company’s outstanding, quantumly supreme AI system? This outfit spends millions on running AI tests which allow the firm’s smart software to perform in an optimal manner in the messaging department. This is “eating the dog food one has prepared.” Think of it as quality testing.
- The AI system, running in the Google Cloud on Google technology is faster than even a quantumly supreme Googler when it comes to generating feel-good platitudes. The technology works well. Evaluate this message in terms of the effectiveness of the messaging generated by Google leadership with regard to the Dr. Timnit Gebru matter. Upper quartile of performance which is far beyond the dead center of the bell curve humanoids.
My view is that there is one positive from this use of smart software to message a partially-developed and not completely educated younger person. The Sundar & Prabhakar Comedy Act has been recycling jokes and bits for months. Some find them repetitive. I do not. I am fascinated by the recycling. The S&P Show has its fans just as Jack Benny does decades after his demise. But others want new material.
By golly, I think the Google ad showing Google’s smart software generating a parental note is a hoot and a great demo. Plus look at the PR the spot has generated.
What’s not to like? Not much if you are Googley. If you are not Googley, sorry. There’s not much that can be done except shove ads at you whenever you encounter a Google product or service. The ad illustrates the mental orientation of Google. Learn to love it. Nothing is going to alter the trajectory of the Google for the foreseeable future. Why not use Google’s smart software to write a sympathy note to a friend when his or her parent dies? Why not use Google to write a note to the dean of a college arguing that your child should be admitted? Why not let Google think for you? At least that decision would be intentional.
Stephen E Arnold, July 31, 2024
How
How
How
How
How
Spotting Machine-Generated Content: A Work in Progress
July 31, 2024
This essay is the work of a dinobaby. Unlike some folks, no smart software improved my native ineptness.
Some professionals want to figure out if a chunk of content is real, fabricated, or fake. In my experience, making that determination is difficult. For those who want to experiment with identifying weaponized, generated, or AI-assisted content, you may want to review the tools described in “AI Tools to Detect Disinformation – A Selection for Reporters and Fact-Checkers.” The article groups tools into categories. For example, there are utilities for text, images, video, and five bonus tools. There is a suggestion to address the bot problem. The write up is intended for “journalists,” a category which I find increasingly difficult to define.
The big question is, of course, do these systems work? I tried to test the tool from FactiSearch and the link 404ed. The service is available, but a bit of clicking is involved. I tried the Exorde tool and was greeted with the register for a free trial.
I plugged some machine-generated text produced with the You.com “Genius” LLM system in to GPT Radar (not in the cited article’s list by the way). That system happily reported that the sample copy was written by a human.
The test content was not. I plugged some text I wrote and the system reported:
Three items in my own writing were identified as text written by a large language model. I don’t know whether to be flattered or horrified.
The bottom line is that systems designed to identify machine-generated content are a work in progress. My view is that as soon as a bright your spark rolls out a new detection system, the LLM output become better. So a cat-and-mouse game ensues.
Stephen E Arnold, July 31, 2024